The Last, Greatest Post About Austin Jackson & BABIP: Part 4 – Variance
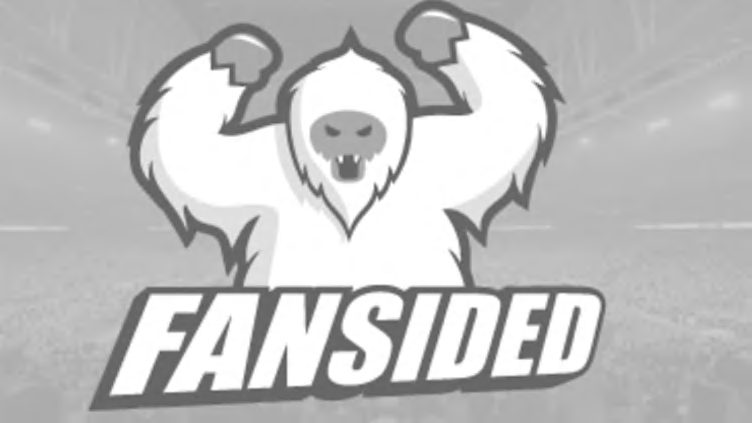
This one is, in fact, the last of the series – attempting to uncover why it is that BABIP is thought of as a less ‘reliable’ skill by statheads, to the extent of alleging that BABIP is really just luck in disguise, and whether BABIP has gotten a bad rap or whether concerns are broadly justified. I will warn you before you progress, this is going to get ‘statty’.
At the root of the discomfort over BABIP as a measure of a hitters(or pitchers) true prowess is the fact that this year’s BABIP doesn’t do a great job of predicting next year’s BABIP. It isn’t so much that BABIP skills don’t exist in theory on either side of the plate but that a high BABIP this year can’t necessarily be taken to mean that the player actually has those skills. He could have been hot, he could have been lucky. But, really, those concerns apply to all metrics as well – is it fair to separate BABIP from the pack?
One way to answer the question is to look at the year-to-year variability for different offensive statistics for individual players to see whether BABIP, or any other stat, seems to show ‘more’ variance than the others. To do that, I’ll need to define a couple of statistical terms: the first is ‘standard deviation’, this is the average amount by which a player’s stat deviates from his career average in one direction or another – I won’t make this piece any more ‘statty’ than it has to be by showing the formula to calculate the thing. So if we see a career batting average of .300 and a standard deviation of .030, that would mean that the player would – in an average year – wind up 30 points off his career average either above or below. A player with a batting average standard deviation of .040 would be noticeably more prone to streaks and slumps, good years and bad. A standard deviation of .000 would mean that the player matched his career average precisely every year. If one stat has a high standard deviation and another a low standard deviation, that means that the second stat is a good deal more reliable.
The next term is ‘coefficient of variation”: that’s a closely related statistical concept, we simply take the standard deviation for that stat and divide it by the player’s career average. So we would convert that standard deviation of .030 to a coefficient of variation of .1 or 10% in order to make comparisons between metrics more relevant. It may not be tremendously informative to see that the standard deviation of a player’s batting average is 3 times the standard deviation of his walk rate, if the walk rate is only .10.
The next step is to find some appropriate players – we want guys with long careers, so many observations to look at, and we also want a sprinkling spread along the BABIP spectrum so we aren’t looking exclusively at the high BABIP guys. I decided to look at this as more of a case study approach and pick a couple of guys with high career BABIPs, a couple of guys close to league average, and a couple of guys way below the curve. To use guys who played fairly close to the modern era, my examples for high-BABIP players are Rod Carew and Derek Jeter. Since guys with average BABIPs are abundant, I went the relevance route and picked our very own Lou Whitaker and Alan Trammell. Pickings were slim for the low-end of the BABIP spectrum, since I wanted guys with long careers and lots of plate appearances. In order to be worthy of that, despite a terrible BABIP, a player would have to really excel in multiple other facets of the game. Two guys that have are Graig Nettles (who excelled in power and defense) and former Tiger Darrell Evans
(who excelled in power and patience).
First of all, here are their career numbers: bear in mind that due to talent and longevity all of these guys are at least in the running for future admission to the Hall of Fame (where thus far only Carew presently resides):
For reference, so far in 2011 the league as a whole is averaging a BB% of .0845, a K% of .1823, an ISO of .138 a batting average of .252, a BABIP of .297 and OBP of .320 and a slugging percentage of .390. Bear in mind that in spite of the drop in scoring following the end of the juiced era, the 1960s and 1970s (and to a lesser extent 1980s) saw less offense than today.
Now take a look at the players’ standard deviations and standard errors:
Which stats seem the least reliable?
If we compare standard errors for BABIP and Isolated Power, walk rates and strikeout rates – all of which are supposedly much more consistent and predictable attributes than BABIP – it is BABIP that looks like the one to bet on. If we start from the knowledge, after a long career, that a player actually does have (or does not have) BABIP skills we don’t see guys that rely on high BABIP numbers to be productive being any flakier or less consistent than guys that rely on a batting eye or biceps – if anying they may be more consistent and reliable. There is nothing wrong, inherently, with trying to build a team around balls in play skills. Nor is there anything wrong, inherently, with putting a guy in the leadoff slot who needs that high BABIP to set the table. The problem is in recognizing, without the benefit of hindsight, whether those skills exist at all.
Exceptional power hitters can have career ISO numbers at or close to double the league average, the same goes for walk rates (take Barry Bonds for example). An exceptional hitter may strike out only half as much as the league average. No batter, no matter how good, will have a career BABIP of .594 – as I mentioned before Cobb has the highest career BABIP at .378. For actually assessing whether the skill exists, we need something that looks not only at year-to-year variability of a metric but also relates the player’s career number to the league average.
So, it’s time for a new term: the ‘confidence interval’. Most statistical analysis is based on making certain assumptions about probabilities and then plugging in estimates in place of true values. Using, for example, Jeter’s career BABIP number and his year-on-year standard deviation as a measure of variability, we can estimate a range (assuming a ‘normal distribution’ for annual stats around that career average) in which we are fairly certain that Jeter’s ‘true BABIP’ will fall. For Jeter specifically, we can be 95% certain that Jeter’s true BABIP falls somewhere in between .350 and .363. .350 is clearly much greater than the league average of .297 so after 16 years in the big leagues we can be pretty darn sure that Jeter actually has BABIP skills (and loads of them). The higher the standard deviation for a statistic is, especially relative to the size of the variable, the wider the confidence interval will be and the more likely that we will be unsure (in a statistical sense) whether the true value is greater than the league average at all. The confidence interval also gets narrower the longer the player’s career has been, after only 5 seasons (if Jeter had exhibited the same career BABIP and standard deviation) we could only say that his true BABIP was between .368 and .345. That would be much wider if we had only, say, two years by which to judge a player.
Of course, the closer a player’s career BABIP number is to league average, and players like Jeter are unusually far from the mean, the harder it is to say anything at all about their skill. If a player with two years of service time had a .350 BABIP in his rookie year and then followed it with a .270 in year two – his career BABIP would be .310, which shows a ‘skill’ along the lines of a Magglio Ordonez, in a statistical sense we could only say that his ‘true’ BABIP was somewhere between .275 and .345 – which could either mean HoF-caliber BABIP skills or a relative lack of BABIP skills that could ultimately cost him a roster spot. Most of the time, hitters and pitchers who have shown some BABIP skills in their first few years (like Porcello or Avila) fit into this category. The variability in their numbers vastly outweighs the possible difference between them and the average pitcher or batter, by the time Porcello is 35 we may be able to see conclusive proof (in a statistical sense) that he has the skill to keep a BABIP 10 or 15 points below average – but by then it will be a little late for the organization to make smart roster decisions.
Statheads should bear in mind, however, that this is also true for other attributes: a player who hits a lot of home runs one year and experience a ‘power outage’ the next is a common phenomenon, and we are equally unable (in a statistical sense) to tell which year’s production is closer to the player’s true talent. It’s just there is more differentiation across players in ‘power’ than there is in ‘BABIP’.
Now: Back to Austin Jackson, who is, afterall the reason this has been researched, written and posted: Jackson does have a limited track record, but he does not fit into that ‘sometimes quite a bit over, sometimes a little under’ class of player for which we have difficulty identifying true abilities. Last year Jackson was waaaaay over league average, this year he is still waaay over (with two fewer a’s) – even given the tiny sample size, we can be 95% confident that Jackson’s true BABIP lies between .356 and .405. So please, don’t expect Jackson to simply ‘regress to the mean’. Jackson isn’t an average player, where BABIP is concerned. Nor is BABIP uniquely unreliable as a measure of skill. The true question is this: If Jackson’s true BABIP is at the lower end of that range (which would be my guess), does he have enough talent in other areas to be a great player?