The Last, Greatest Post About Austin Jackson & BABIP: Part 4 – Variance
By Chris Hannum
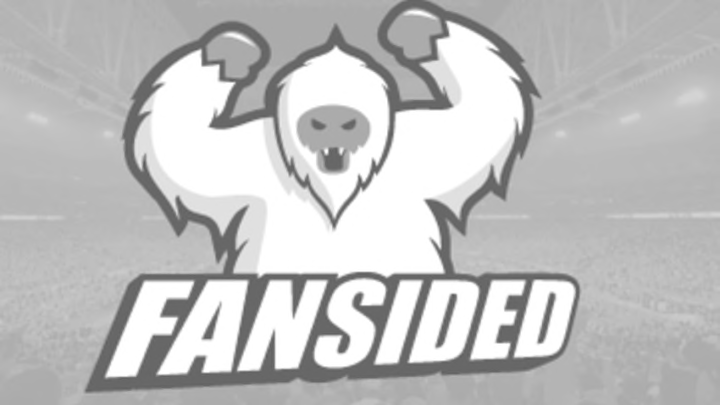
This one is, in fact, the last of the series – attempting to uncover why it is that BABIP is thought of as a less ‘reliable’ skill by statheads, to the extent of alleging that BABIP is really just luck in disguise, and whether BABIP has gotten a bad rap or whether concerns are broadly justified. I will warn you before you progress, this is going to get ‘statty’.
At the root of the discomfort over BABIP as a measure of a hitters(or pitchers) true prowess is the fact that this year’s BABIP doesn’t do a great job of predicting next year’s BABIP. It isn’t so much that BABIP skills don’t exist in theory on either side of the plate but that a high BABIP this year can’t necessarily be taken to mean that the player actually has those skills. He could have been hot, he could have been lucky. But, really, those concerns apply to all metrics as well – is it fair to separate BABIP from the pack?
One way to answer the question is to look at the year-to-year variability for different offensive statistics for individual players to see whether BABIP, or any other stat, seems to show ‘more’ variance than the others. To do that, I’ll need to define a couple of statistical terms: the first is ‘standard deviation’, this is the average amount by which a player’s stat deviates from his career average in one direction or another – I won’t make this piece any more ‘statty’ than it has to be by showing the formula to calculate the thing. So if we see a career batting average of .300 and a standard deviation of .030, that would mean that the player would – in an average year – wind up 30 points off his career average either above or below. A player with a batting average standard deviation of .040 would be noticeably more prone to streaks and slumps, good years and bad. A standard deviation of .000 would mean that the player matched his career average precisely every year. If one stat has a high standard deviation and another a low standard deviation, that means that the second stat is a good deal more reliable.
The next term is ‘coefficient of variation”: that’s a closely related statistical concept, we simply take the standard deviation for that stat and divide it by the player’s career average. So we would convert that standard deviation of .030 to a coefficient of variation of .1 or 10% in order to make comparisons between metrics more relevant. It may not be tremendously informative to see that the standard deviation of a player’s batting average is 3 times the standard deviation of his walk rate, if the walk rate is only .10.
The next step is to find some appropriate players – we want guys with long careers, so many observations to look at, and we also want a sprinkling spread along the BABIP spectrum so we aren’t looking exclusively at the high BABIP guys. I decided to look at this as more of a case study approach and pick a couple of guys with high career BABIPs, a couple of guys close to league average, and a couple of guys way below the curve. To use guys who played fairly close to the modern era, my examples for high-BABIP players are Rod Carew and Derek Jeter. Since guys with average BABIPs are abundant, I went the relevance route and picked our very own Lou Whitaker and Alan Trammell. Pickings were slim for the low-end of the BABIP spectrum, since I wanted guys with long careers and lots of plate appearances. In order to be worthy of that, despite a terrible BABIP, a player would have to really excel in multiple other facets of the game. Two guys that have are Graig Nettles (who excelled in power and defense) and former Tiger Darrell Evans
(who excelled in power and patience).